The Advantages and Disadvantages of Using Synthetic Data as a Sample Source
In the realm of market research and data analysis, synthetic data has emerged as a fascinating and increasingly viable option. Synthetic data, generated through algorithms and simulations, can mimic real-world data with high fidelity. While it offers several compelling advantages, it also presents unique challenges and potential drawbacks. This blog explores the benefits and limitations of using synthetic data as a sample source.
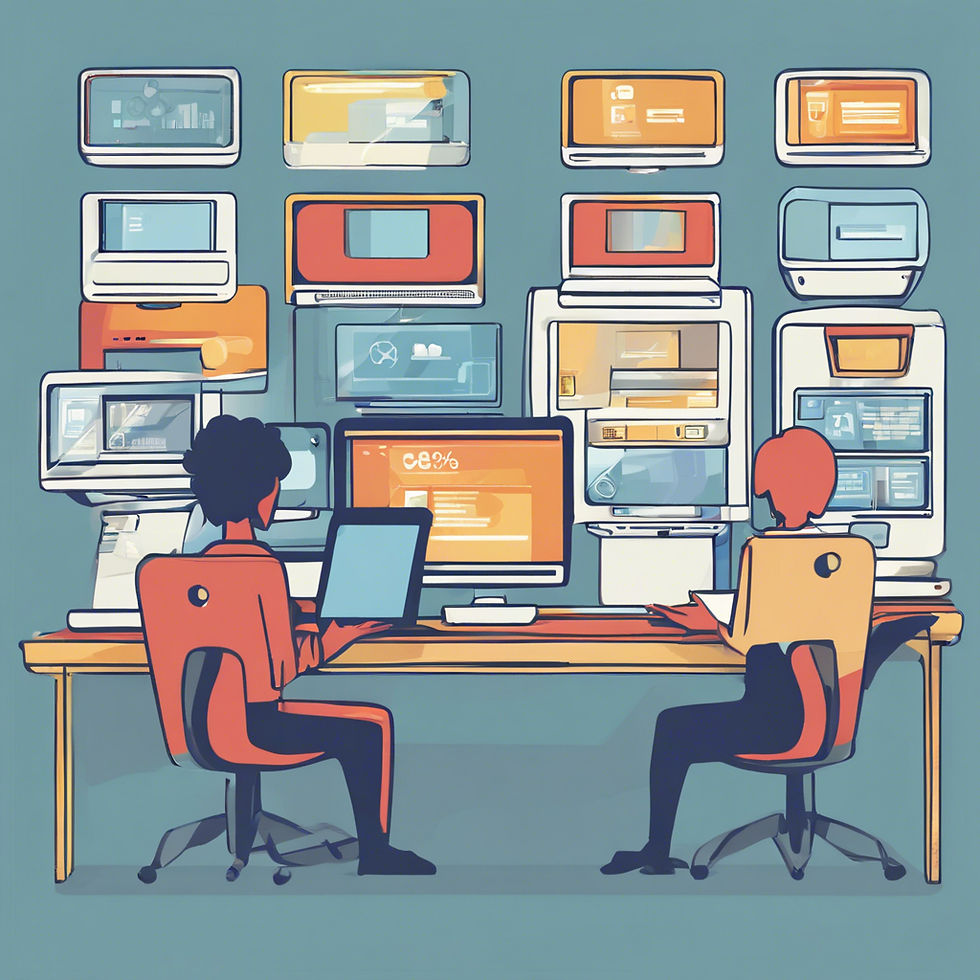
Advantages of Using Synthetic Data
1. Privacy Preservation
One of the most significant advantages of synthetic data is its ability to preserve privacy. By creating data that mimics real-world patterns without containing actual personal information, organisations can conduct detailed analyses without risking data breaches or compromising individual privacy. This is particularly crucial in sectors like healthcare, finance, and social sciences, where data sensitivity is paramount.
2. Cost-Effectiveness
Generating synthetic data can be more cost-effective than collecting real-world data. Traditional data collection methods often involve significant expenses related to surveys, incentives, and data entry. Synthetic data, on the other hand, can be produced at a fraction of the cost once the initial algorithm and model are in place. This can make market research more accessible, especially for small and medium-sized enterprises.
3. Data Availability and Scalability
Synthetic data can be generated on demand and at scale. Researchers and analysts are not limited by the availability of real-world data, which can be scarce or difficult to obtain. This flexibility allows for extensive experimentation, hypothesis testing, and model training without the constraints of data scarcity.
4. Bias Reduction
By carefully designing synthetic data, it is possible to reduce or eliminate biases inherent in real-world data. This can lead to more accurate and fair analysis, particularly in areas where historical data may be tainted by systemic biases. Synthetic data allows researchers to control for specific variables and create balanced datasets that reflect desired scenarios.
Disadvantages of Using Synthetic Data
1. Lack of Authenticity
The primary drawback of synthetic data is its lack of authenticity. No matter how advanced the algorithms, synthetic data is ultimately a simulation and may not capture all the nuances of real-world behaviour. This can be a significant limitation when detailed and context-specific insights are required.
2. Validation Challenges
Validating synthetic data is inherently challenging. Ensuring that synthetic data accurately represents real-world patterns and distributions requires rigorous testing and validation. Any discrepancies can lead to flawed conclusions and undermine the credibility of the research. Establishing robust validation frameworks is essential but can be resource-intensive.
3. Algorithmic Limitations
The quality and reliability of synthetic data are heavily dependent on the algorithms used to generate it. If the underlying models are flawed or biased, the synthetic data will inherit these issues. Continuous improvement and monitoring of the algorithms are necessary to maintain the integrity of the synthetic data.
4. Regulatory and Ethical Concerns
While synthetic data mitigates many privacy concerns, it is not entirely free from regulatory and ethical considerations. The creation and use of synthetic data must still adhere to legal frameworks and ethical guidelines, particularly in how it is generated and applied. Misuse of synthetic data can still lead to ethical dilemmas and potential legal repercussions.
Conclusion
Synthetic data represents a powerful tool in the arsenal of modern market research and data analysis. Its advantages in terms of privacy preservation, cost-effectiveness, and scalability make it an attractive option for many applications. However, researchers must be mindful of its limitations, particularly regarding authenticity, validation challenges, and ethical considerations.
Incorporating synthetic data into research methodologies requires a balanced approach, leveraging its strengths while acknowledging and mitigating its weaknesses. As technology and algorithms continue to advance, the role of synthetic data in market research is likely to expand, offering new opportunities and challenges in equal measure.
By understanding both the potential and
4o
Include examples of recommended synthetic data sources
ChatGPT
The Advantages and Disadvantages of Using Synthetic Data as a Sample Source
In the realm of market research and data analysis, synthetic data has emerged as a fascinating and increasingly viable option. Synthetic data, generated through algorithms and simulations, can mimic real-world data with high fidelity. While it offers several compelling advantages, it also presents unique challenges and potential drawbacks. This blog explores the benefits and limitations of using synthetic data as a sample source and recommends some reliable synthetic data sources.
Advantages of Using Synthetic Data
1. Privacy Preservation
One of the most significant advantages of synthetic data is its ability to preserve privacy. By creating data that mimics real-world patterns without containing actual personal information, organisations can conduct detailed analyses without risking data breaches or compromising individual privacy. This is particularly crucial in sectors like healthcare, finance, and social sciences, where data sensitivity is paramount.
2. Cost-Effectiveness
Generating synthetic data can be more cost-effective than collecting real-world data. Traditional data collection methods often involve significant expenses related to surveys, incentives, and data entry. Synthetic data, on the other hand, can be produced at a fraction of the cost once the initial algorithm and model are in place. This can make market research more accessible, especially for small and medium-sized enterprises.
3. Data Availability and Scalability
Synthetic data can be generated on demand and at scale. Researchers and analysts are not limited by the availability of real-world data, which can be scarce or difficult to obtain. This flexibility allows for extensive experimentation, hypothesis testing, and model training without the constraints of data scarcity.
4. Bias Reduction
By carefully designing synthetic data, it is possible to reduce or eliminate biases inherent in real-world data. This can lead to more accurate and fair analysis, particularly in areas where historical data may be tainted by systemic biases. Synthetic data allows researchers to control for specific variables and create balanced datasets that reflect desired scenarios.
Disadvantages of Using Synthetic Data
1. Lack of Authenticity
The primary drawback of synthetic data is its lack of authenticity. No matter how advanced the algorithms, synthetic data is ultimately a simulation and may not capture all the nuances of real-world behaviour. This can be a significant limitation when detailed and context-specific insights are required.
2. Validation Challenges
Validating synthetic data is inherently challenging. Ensuring that synthetic data accurately represents real-world patterns and distributions requires rigorous testing and validation. Any discrepancies can lead to flawed conclusions and undermine the credibility of the research. Establishing robust validation frameworks is essential but can be resource-intensive.
3. Algorithmic Limitations
The quality and reliability of synthetic data are heavily dependent on the algorithms used to generate it. If the underlying models are flawed or biased, the synthetic data will inherit these issues. Continuous improvement and monitoring of the algorithms are necessary to maintain the integrity of the synthetic data.
4. Regulatory and Ethical Concerns
While synthetic data mitigates many privacy concerns, it is not entirely free from regulatory and ethical considerations. The creation and use of synthetic data must still adhere to legal frameworks and ethical guidelines, particularly in how it is generated and applied. Misuse of synthetic data can still lead to ethical dilemmas and potential legal repercussions.
Recommended Synthetic Data Sources
To maximise the benefits of synthetic data while minimising its drawbacks, it is essential to use reliable and well-regarded synthetic data sources. Here are some recommended sources:
Syntho: Syntho provides high-quality synthetic data solutions, focusing on privacy preservation and data utility. Their platform allows users to generate and validate synthetic datasets tailored to specific use cases.
Hazy: Hazy specialises in synthetic data generation for financial services and other sectors requiring stringent data privacy. Their AI-driven platform ensures that synthetic data maintains the statistical properties of real-world data.
MOSTLY AI: MOSTLY AI offers a comprehensive synthetic data platform that generates realistic and privacy-compliant datasets. Their tool is widely used in industries such as banking, insurance, and healthcare.
Synthea: Synthea is an open-source synthetic patient generator that produces realistic healthcare records. It is particularly valuable for research and development in the healthcare sector, providing synthetic data that mirrors real-world clinical scenarios.
YData: YData provides tools for generating synthetic data that are particularly useful for machine learning applications. Their platform focuses on ensuring data quality and utility, helping users create datasets that are both diverse and representative.
Conclusion
Synthetic data represents a powerful tool in the arsenal of modern market research and data analysis. Its advantages in terms of privacy preservation, cost-effectiveness, and scalability make it an attractive option for many applications. However, researchers must be mindful of its limitations, particularly regarding authenticity, validation challenges, and ethical considerations.
Incorporating synthetic data into research methodologies requires a balanced approach, leveraging its strengths while acknowledging and mitigating its weaknesses. By utilising reputable synthetic data sources like Syntho, Hazy, MOSTLY AI, Synthea, and YData, researchers can harness the benefits of synthetic data while ensuring data quality and compliance with ethical standards. As technology and algorithms continue to advance, the role of synthetic data in market research is likely to expand, offering new opportunities and challenges in equal measure.